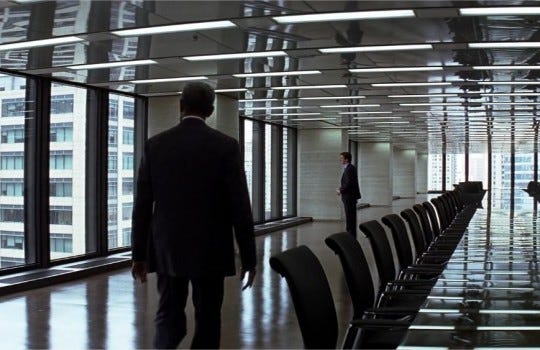
There was a knock on the door. It was the club’s chief scout. He had set some time up with the general manager to discuss a potential transfer target, a roaming central midfielder with an eye for arriving late in the box.
GM: “Come on in.”
******Disclaimer: OK, look, I have never worked in a football club, and I know there are going to be a million things wrong with this scene. This setup may be far too simple, or far too complicated, the dialogue is purposefully -shall I say- direct at times so as to illustrate the concepts. If I have fun with any of it, it is not meant to be caricature of any of these experts. I’ve taken liberties with the responsibilities of the individuals, left out others (the manager is mostly absent for example). And you might notice a hilarious turnaround time for a data project. Just kinda roll with it if you can. There are some points that I think are worth making even if some of this is clunky******
GM: “Come on in.”
The GM is feeling a bit energized by all of this. The scout hasn’t even sat down when…
GM: So…by how many goals will this player improve our team next season and over the full term of his contract?”
Scout: (slightly confused) Hmm, well, I.. Look I’ve got his scouting report right here that we can talk through—
GM: What should we expect the improvement in goal difference to be though?
Scout: Well he assisted 6 goals last year, and scored—
GM: He’s a midfielder though, surely he does other things to help the team win games? I want to know about this total contribution to the team’s results, not just his goal scoring and assists.
Scout: Yea, I know, but you said goals. Look, I’m prepared to tell you all about the player’s strengths and weaknesses, his style of play, his first touch, is work rate, what’s clear watching the tape and what I’ve heard from his coaches and my other contracts in the league… I’m not sure you can just put an exact goal value on a midfielder’s contribution like that. Soccer is hard, and I’ve put a lot of work into finding a player that suits the manager’s needs and the team’s play style.
GM: Of course it is hard, that’s why I value your work so much. Do me a favor, go back through the report and distill it all down and come back to me with your best estimate of how he’s going to impact the team’s overall performance next year .. in terms of goal difference. Humor me. No wrong answers today.
Scout: Sure thing, boss.
Scout leaves his office and the Data Analyst walks in, oh God it looks like he has a spreadsheet already up. The GM subconsciously reaches for a mug of coffee that he had sworn off only last month.
GM: By how many goals will this player improve our team next season and over the full term of his contract?”
Data Analyst: *smirks* well, there’s more to the game than goals. I collect a variety of metrics: *clicking through slides* Cross to throughball ratio, expected goals, expected assists and xG chain, and there’s a player’s xPass score from the expected passing model, and we can look at his defensive actions per possession, and his aerial and ground duel rates. It’s important to strip out penalties. +/- is problematic for many reasons, but I can share that if you want to see it. I can show you some radar char—
GM: Stop. By how many goals will this player improve our team’s overall performance next season and over the full term of his contract?”
Data Analyst: Well, I don’t know exactly, soccer is hard. That’s why I have these radar char—
GM: Of course it is hard, that’s why I value your work so much. Come on now. You can rattle off any number of advanced analytics metrics but you can’t tell me the headline takeaway of why we should sign this player? It must be that he will improve the team’s success on the field, namely by improving our goal scoring or goal conceding, right? Try again, and we’ll get back together.
Data Analyst leaves his office and the Scout walks back in.
Scout: OK I thought about what you were saying and while soccer is hard, I have years of experience playing, coaching, and evaluating players and here’s what I think. Last year our central midfielder was below average. To my eye, he lacks accuracy in passing, his legs are going a bit, and he’ll be a year older so he doesn’t cover as much ground as he used to. When he does attempt tackles, he wins the ball well enough, and he takes free kicks well, but we could do much better. This new guy we want you to sign passes the ball better and quicker, he covers more ground to disrupt attacks, and he gets into the box with late runs to help his team score. He should help us advance the ball to our attackers more often, and disrupt opposition attacks from entering our third of the pitch with more efficiency. Above all, he’s only 23 years old and his peak years are in front of him. These things are really hard to judge, but in my estimation we might concede 2-3 fewer goals this year with him, and score 3 more due to his contributions in buildup and attack, and this will only improve in the coming years. I think he could be a real star, adding 7-8 goals a year for us compared to an average player in the same spot, once he gets settled in.
GM: OK I’m not convinced yet. After all, as you said, it’s not easy to distill a scouting report down into a number like this, but I think I understand what you’re saying. Thank you for packaging it together this way. (shouting) Data Analyst, come on in!
Data Analyst: Boss, I thought about what you were asking for — some way to measure the total contribution of a player to his team in terms of goal difference, and here’s what I came up with: I fed decades of match data into a machine learning algorithm to teach it how to estimate for any moment in a match the probability of a team scoring on its current possession and the probability of that same team conceding on its next possession. I then assigned the differences in those probabilities from one moment to the next to all the events in the data (passes, shots, dribbles, tackles, clearances). I allocated the valued contributions calculated for each of those events (whether they be positive or negative contributions) to the players involved and out popped an estimate of how each player in the league has changed his team’s goal scoring and conceding probabilities with each touch he’s made. It effectively shows how many “goals” a player has contributed to his team looking at all his touches, not just plays that actually resulted in goals or assists, shots or shot assists! I probably need to do some fine-tuning, but it says this player has added between 4-5 goals worth of value per season to his team the last couple of seasons! With some more time I can show you how exactly he might contribute.
GM: That’s wild, that is exactly the sort of thing that I’d want to know about a player we’re going to splash the cash for. But the machine spat out that number without knowing the player’s age, or watching a second of tape on the player or talking to his coaches or teammates or other scouts in the league?
Data Analyst: Yea! Isn’t that incredible!
GM: For sure, it’s impressive. But you trust it then?
Data Analyst: Well, I mean… yea?
GM: OK, well soccer is hard, so I don’t see how we could trust this output on its own, plus that only tells me how the machine has rated his past contributions. The past is the past and we’re not about to pay for the past. What we really care about are his future contributions to this team, right? How will he perform in this environment, vs the team and league he came from? Do we expect him to improve during his time here? Sounds like you’re on the right track, but both of you need to get together and try to work out between the two of you, what we have and don’t have, what we know and what we don’t. In the mean time, I’ll work to firm up an overall template/approach we can use in these situations going forward, and I’ll need your help in building that out as well. Remember, we have to close a +15 goal differential gap this year, and so as a starting point, we need to make some moves that we can confidently say move us towards that figure.
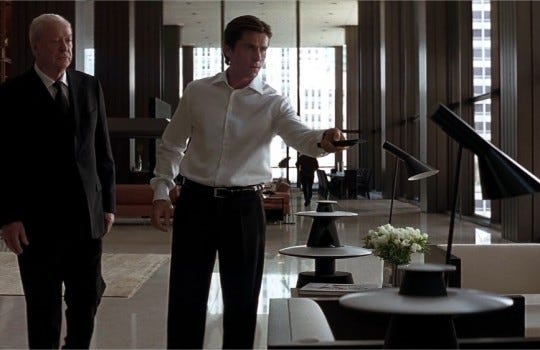
What have we learned?
We will return to this story several weeks from now, but I think it’s worth noting that if we assume the GM is correct to make his decisions based on the marginal goal difference impact of his options, then clearly neither the scout nor the data analyst brought enough to the table on the first pass to meet his needs. That said, as I see it, the data analyst carries the heavier burden in this failure, because one selling point of analytics is that it can quantify the game into objective digestible soundbites like this for a decision maker. It’s certainly not the most significant selling point for analytics, but I could understand the frustration that the general manager feels. It’s one thing for the scout to have struggled to put a number on it, but the GM was hoping to get a more tangible data point from his data analyst and was presented (at first) with a grab bag of somewhat opaque insights (even if we assume they are predictive and accurate).
Conjecture on past grievances with data analytics
If the scout had been allowed to continue his first conversation with the GM, at least he would have been able to articulate in footballing terms why it is he recommended signing the player, what he likes and a general degree of how good the player is. While he might be prone to various biases that the data analyst is more systematically able to guard against and while he would not have delivered on a robust measurable Key Performance Indicator that the GM was asking for, he would have communicated largely what people talk about when they talk about player evaluation. Further, he could also support his ideas with the assertion that he had literally watched all those hours of film, he had done the interviews.
The data analyst on the other hand, for all his additional benefits around avoiding biases, the delivery of (let’s assume) proven predictive analytics, and the production of (hopefully) beautiful visualizations, cannot say the same. If he’s not using all of his data models in a way that transforms his findings into the “data format” that the GM is craving to consume for his decision making process, it’s a massive disappointment. And I say this as an analytics convert/disciple and as you’ll see going forward, someone who sees analytics as the foundation of a good player projection process. There are of course many other benefits to using this portfolio of analytics findings from the data analyst: data is cheaper than live scouting and analytics might provide a great first cut or a filter on the front end of the process to create a shortlist toward which the scout can be directed. He might be able to help the GM avoid large mistakes (e.g. he might identify that a target forward’s recent run of form is as much down to chance as it is skill). Ultimately, when it comes to supporting an important decision by the general manager, someone who is expecting something quantifiable to come from the quantitative analyst, the analyst must deliver insight that aligns with the question at hand, otherwise it’s easy to sympathize with the GM’s frustrations, or even his skepticism.
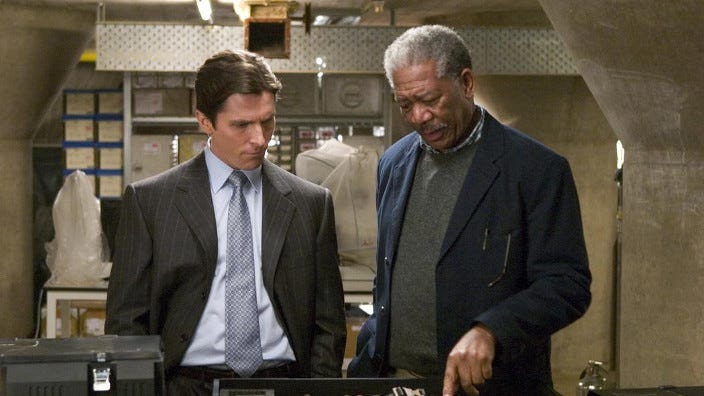
Make it impossible to ignore
Importantly, once the data analyst does align the form of his findings with the GM’s expectations, namely denominating his outputs into the unit of account the GM is using to support decision making, his input suddenly becomes impossible to ignore. If a GM is asking “what is the marginal contribution to goal difference I can expect if I sign this player?” and the data analyst answers this question directly (“6 goals over the course of a season”), and then briefly explains how the model works and potentially the areas of the game within which he expects the player to add such value, it is persuasive. Further, any answer from the scout that is wildly different from 6 goals, will need to be explained, and there will often be good explanations for such differences. In my mind, this is exactly one of the key roles that analytics should play in player recruitment. Having aligned the two languages for value, analytics is no longer a “moreover” the GM adds into the “go/no-go” decision point. It is now fundamental to him answering the original question at hand, and it is now fundamental into the decision making within soccer operations. While the scout’s input now exists as an important input into the architecture of “marginal goal difference,” the data insights might be said to provide the very foundation of the player evaluation itself. You could visualize this as a stack of information with the weighty quant data on the bottom and qualitative data sat upon it, or you could walk the various inputs across a screen from hard data to ultimate recommendation in a handy format used by finance departments that I’ll explore in a later post.
I’ll quickly note again, that the scenario I’ve laid out is an unfair representation of “football analytics,” more specifically of what has traditionally been available publicly. We know for instance that at Liverpool, Ian Graham’s division does or did exactly this sort of “one single currency” approach to player recruitment. And we know, that in the last few years, “expected possession value” (EPV) models have become more and more available in the public sphere, which are the exact sort of advanced models that the data analyst in the above parable generates unrealistically quickly between his two meetings with the GM. I’ll cover this in more detail soon enough, but it is my belief that EPV models add the secret ingredient (a “unit of account”) that unlocks an already otherwise very rich soccer event data set for further use in the industry.
Also, it would be unfair to characterize all of recent criticisms of analytics in soccer as good faith arguments. Plenty of the most newsworthy examples were embarrassing ill-considered rants by the ignorant, and I won’t get into that.
All-in, my point is not to smear the work of public soccer analytics. It is to empower it. With the rise of EPV type metrics, one of which I’m particularly familiar with and will explore further in later posts, if you are an advocate of an expanded use of analytics in soccer (and you don’t have to be to read these newsletters - that’s fine), there is a profoundly great opportunity to maximize the use of analytics in the player recruitment process simply by 1) advocating for the “marginal goal difference” framework to be used in decision making processes — this bit I don’t find controversial and is mostly agnostic to the use of analytics — and then 2) aligning the outputs from the football analytics community directly onto this decision making framework, using the exact quantifiable language, so as to make them impossible to ignore.
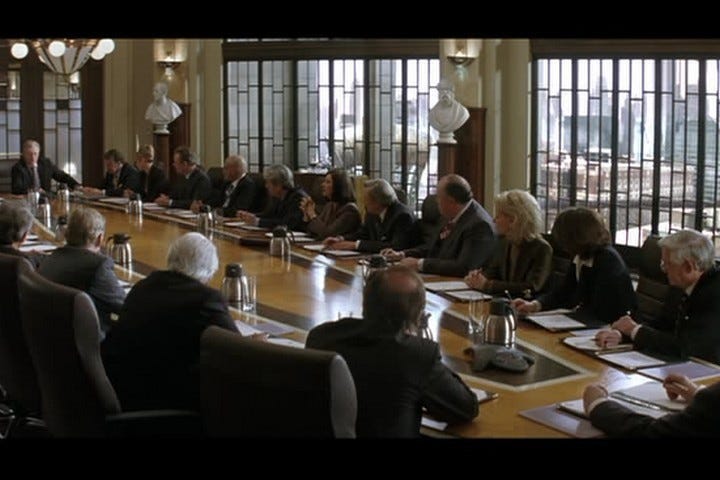
But how?
Distilling the myriad of complexities of fluid and dynamic systems down into a single projection or equation for the purposes of a “go / no-go” decision is no small task, but it is exactly the task that corporate finance functions, hedge funds, and investment analysts have faced so long as they have existed. And we know how they go about it. These textbook principles are taught to every business major in higher level education. The next post will briefly explore the most common sophisticated framework so as to provide a summary of its basic concepts, then after that, we’ll borrow it for soccer purposes with the aim of building the best possible player recruitment process.
Post scriptum: The right question
In today’s example the General Manager was sort of handed the golden question deus ex. And accordingly, in the analysis above, the scout and the data analyst (mostly the latter) unfairly bear the brunt of the uh.. banter? But obviously, an important point to reckon with is that if the GM doesn’t ask the right question to begin with, this discussion can go in any number of directions, and it won’t really be clear whether the destinations are satisfactory or not. Things may often work out. Clubs with good scouts and good analysts do generate good insights. To the extent the decision makers really internalize those insights and use them to sign players, that’s great and directionally, it’s a win. It’s likely sustainable. But even in an environment like that, I would allege there’s many a slip 'twixt the cup and the lip. The value of any given decision is not binary, in the sense that not all good decisions are equally good, nor are bad ones equally bad. This spectrum of values feels like it’s the first bit of information to be lost in the transfer from insight to decision if the correct question is not posed to begin with. The purpose of “Absolute Unit” then is to deploy a framework or interface such that the great insights that scouts, analysts, coaches, players etc generate are properly valued and connected to important decision making.
Thanks again for reading. Subscribe and share with a friend.