Reducing noise with pro-forma adjustments
Step 2: Use consensus soccer analytics insights to prime your soccer accounting records for safe use going forward into the unknown.
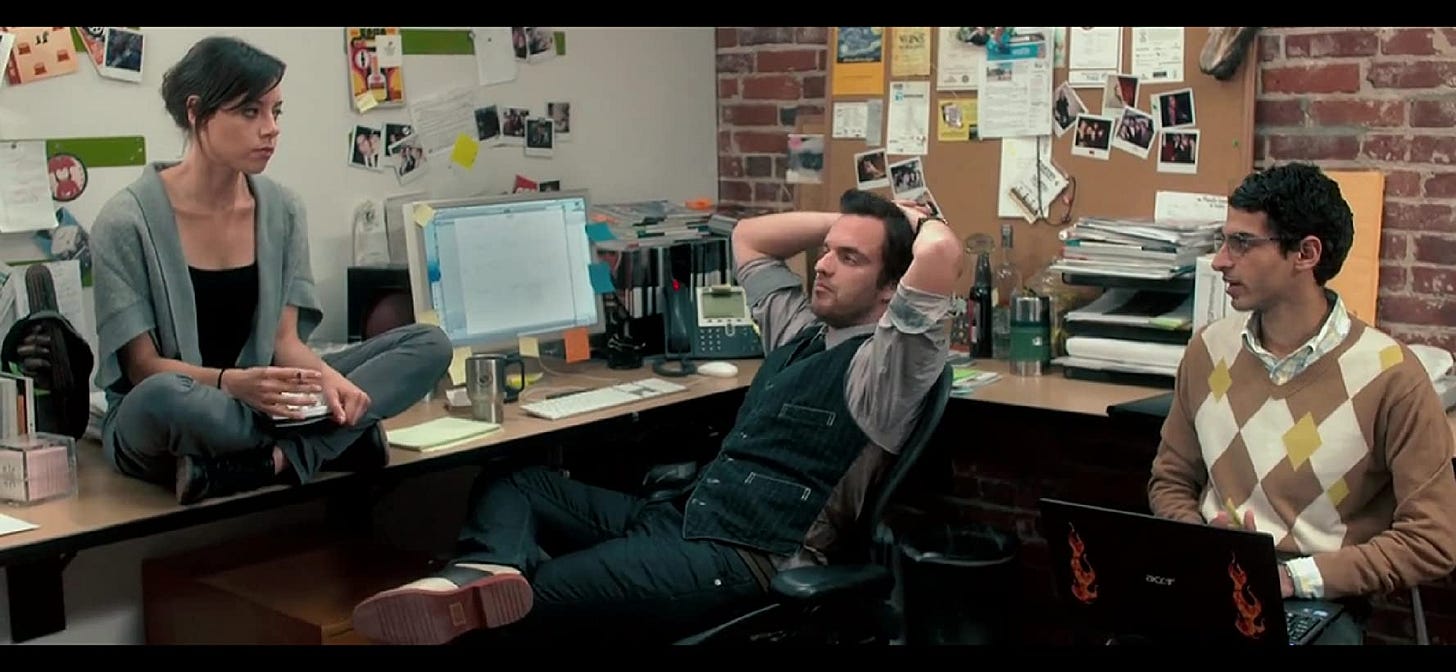
Previously On “Absolute Unit”
The financial world estimates the “value” of an asset based on (i) the projected future cash flows it generates, which are then discounted to price it relative to the amount, risk, and timing of similar assets' cash flows using (ii) the concept of a “required rate of return.” To (i) project future cash flows the basic steps we agreed on were:
Obtain relevant and reliable accounting records of historical results, which are (1a) denominated in the unit of value that you care about (in our case “marginal goal difference contribution”).
Adjust the historical results for noisy, non-recurring items to create “pro-forma historical results.”
Take those pro-forma historical results and project them forward into the future in some kind of documented, evidenced-based manner based on the information publicly available.
Layer in any proprietary information you may have related to the thing being valued.
Apply individual judgments not already included above.
Where we left off in the last post, is that in the analogous soccer world, we have finally completed step 1: we grabbed all the relevant historical results, every action our target player signing has performed in the dataset. Then, we used an EPV model to assign a value to each of those actions, the value measured as the change in probabilities of his team scoring and conceding over a two possession sequence, bringing these soccer accounting records nearly on par with financial accounting records. That means we have pretty good handle on how valuable this target player has been to his team(s) in the past. But we are not about to sign him to a contract (let alone pay a fee to another club to terminate his current contract so that we can then sign him to a new contract) based on his past performance. If we, the GM (I’m mixing up the narrative voices here) are going to improve the team’s goal difference by +27 next year, vaulting the team from 6th to 4th to claim the last spot in the Continental Cup, than we must make this decision based not on his past performance, but on an expectation of his future performance to our team.
If we’re going to develop an expectation about the player’s future performance to our team, and if we’re going to start by anchoring the projection in the player’s historical performances, then we have to do some pruning around the edges of his historical performances, to strip out things that definitely happened — we’re not saying they didn’t — but that aren’t a helpful representation of what may lay ahead, and we’ll call these surgical maneuvers “pro-forma adjustments.”
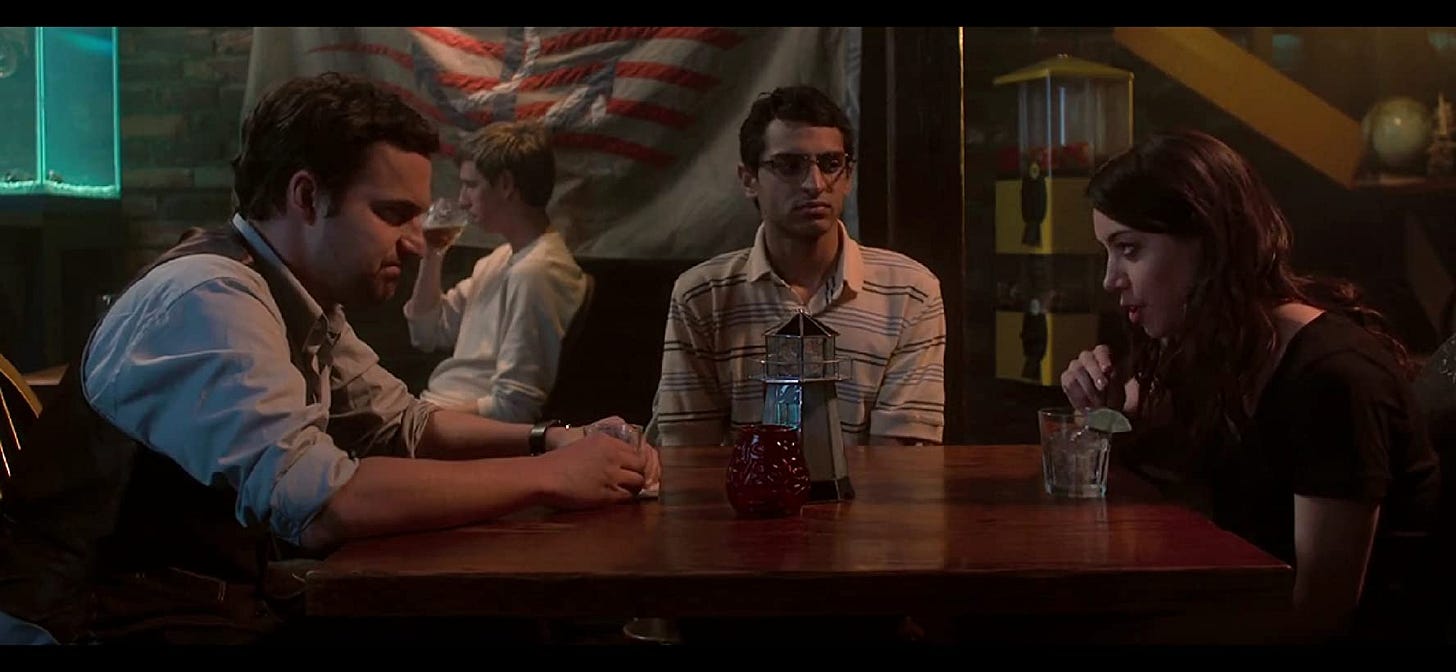
Pattern of Play
Soccer is a fluid and dynamic game, but there are certain parts of the game not like the rest, parts where the play slows down and where the natural dynamic that exists for most of the game where players are in constant movement to progress the ball, to show for the ball or to remove cover for a teammate who is on the ball, comes to a halt. This natural “run of play” state is one in which the degree to which a player is involved in his team’s possessions matters — the degree to which he is recorded in the accounting records as finding passes and playing passes -- carries some signal about his ability to continue to do so in the future. But in the moments where this natural state of play halts, the accounting records begin to pick up on more exogenous variables, and not the natural contributions that flow out of a player in the course of a game.
For instance, when a penalty is awarded and a player takes a shot from the penalty spot, he has not through the natural course of the fluid system, a) carved out an opportunity for himself, b) successfully managed to get a shot off, and then c) put it past the keeper. Instead he has been anointed ahead of time by the manager to take all the penalties. If you visualize the game’s records on an animated pitch, he just sort of teleports to the spot and takes his chance. You don’t want your accounting records teleporting. You could imagine then that if you’re scouting said player, but you already have a good penalty taker on your team, that the past contributions of the target player around penalty kicks (though clearly present in the data) are not indicative of his potential future contribution to your team. Penalty kicks should be removed via pro-forma adjustment. We shouldn’t throw them out entirely — stash them somewhere because we might need them later in Step 3 or 4 — but we don’t want them impacting our “run of play” player projections.
Similarly, when a direct free kick is awarded 20-25 yards from goal, the player who stands over the ball, ready to shoot on goal or create an opportunity for a teammate via delivery into the box, has also himself not “shaken a defender” or “found space” or “received the ball,” nor does he “pick out a pass” or “get off a shot” on his subsequent dead ball attempt. For similar reasons as the above, these accounting records should be stricken or at the very least quarantined from the data as part of our Pro-forma adjustments in Step 2. We want the good stuff. The open play stuff.
You may already have a penalty kick taker or free kick taker on your team, or the target player might take on that role, but your team might find itself experiencing far fewer penalty kick or free kick opportunities than the target player’s current team. These things will need to be projected wholly separate from the target’s player ability to increase his team’s chances of scoring from open play. Same goes for corner kicks. In general, when forecasting a team’s performance and managing towards an objective of “goal difference,” the GM should project his team’s open play expected goal difference, including its ability to win or concede penalties, win or concede set pieces, and win or concede corners (each of these having an impact on the likelihood of scoring). And then separately it should project it’s teams marginal expected goal difference from penalties, set pieces, and corners over and above the expected amounts “won” in the open play forecast. As an example, you want to include the action that wins the corner and therefore caps the possession value off at the 2% corner kick, but not include the subsequent kick itself, which could be a zero (or worse), or an 50% action if a header is won in the six.
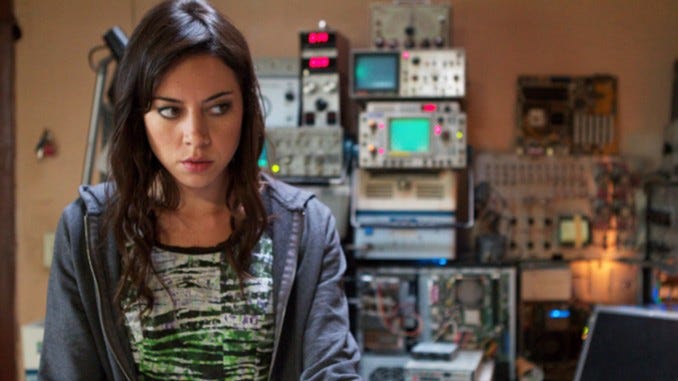
Fluky non-recurring events
There is also an argument to remove other rare actions to which the model attaches high values (whether positive or negative) in the historical records, especially if the target player you’re evaluating is short on minutes played. I don’t have an exact answer for this dilemma, but consider how you might deal with a central midfielder who only plays, let’s say 450 minutes in a season, but finds himself on the end of a single goal mouth scramble and is able to knock a goal in from a yard out — or a player who’s only played say 450 minutes in a season, but in a goal mouth scramble in front of his own goal, he shanks a clearance and it goes straight up or backwards and is then headed home by the opposition. Such single events in the accounting records may well have exceedingly high numbers attached to them, given their proximity to the goals and the fact that the actions themselves did have significant changes on the goal scoring probabilities of the team, but they may not be representative of the player’s future marginal goal difference contribution prospects. If you extrapolate that players 450 minutes out to 3000 minutes over the course of a season, he’s not going to bag 7 goal-mouth scramble goals, nor be responsible for 7 nightmares. Consider excising moments these from the data via pro-forma adjustments before moving onto your projections.
You should also consider adjusting out and quarantining minutes where a target player is playing at uneven strength, with one of the teams having received a red card. With many seasons of data, this type of thing might come out in the wash, but again if you are looking at limited minutes (limited minutes is a problem in general for projections), you would want to break out any disproportionate amount of time spent where the player’s team is down a man, up a man, etc. This same concept goes for if a player has played a disproportionate number of minutes home or away. If you’re targeting a player with 3 or 4 seasons worth of data, this will likely not be an issue.
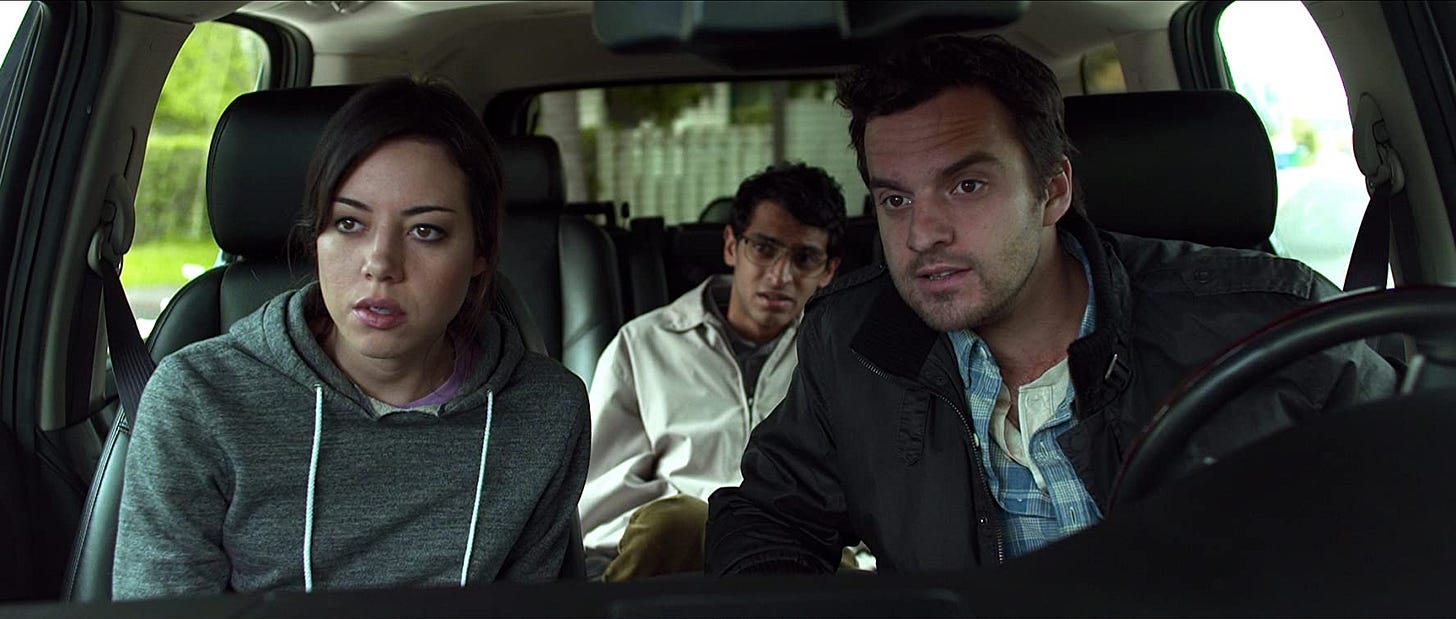
Pro-forma adjustments already contemplated by ASA’s “Goals Added”
The trouble with finishing
The oldest and most obnoxious soccer analytics fight in existence has to do with “finishing skill.” If you’ll indulge me for a moment, the basics are:
Because of how most people experience soccer (by watching it), it’s intuitive to most that the best forwards in the world are the ones who are able to make their opportunities “count” the most — that the best forwards convert shots into goals at higher rates than other forwards.
This intuition is mostly refuted by the fact that once you control for shot location and other variables, the rate at which nearly any forward in an important professional league scores goals above the rate you would expect him to score based on the shooting opportunities he experiences, regresses to the mean HARD over the medium and long term.
This is partly because shots are rare and goals are even more rare (and so any sort of measured finishing rates are subject to an immense amount of noise from small sample sizes in the short term), and partly because the absolute worst “finishing” forwards are weeded out before they reach the highest professional ranks.
Now, the intuition that Robbie Keane is a better finisher than me, someone who does not play professional soccer, is correct, which is why when the analytics findings above (i.e. finishing regression) are sometimes framed/straw-manned as “finishing skill does not exist,” vigorous shit-housing arguments ensue between people who like soccer and who have opinions about it on both sides of the line.
For the record, finishing skill exists. It is real. It is also very difficult to observe in the numbers over the short and medium term. Even more importantly, it actively clouds those numbers unless it is explicitly removed or “nerfed” or otherwise dealt with. In fact, is so difficult to observe and it so actively clouds the data that when forced to use mental heuristics to go about our days, we’re almost always better off assuming something false, that finishing actually does not exist rather than flailing around helplessly trying to measure it and scout for it.
This matters also for our unit-infused accounting records. In an expected possession value model that logs the change in probability of a team scoring moment to moment, because soccer is so hard and goals so scarce, the change in probability from the moment a shot is attempted to the ultimate resolution of a goal or a turnover can be so large as to overwhelm the change in goal probability attached to all other events in the data.
As an example, if we’re applying an EPV model focused for simplicity on just the probability of the team in possession scoring, and our playmaker picks up the ball in the left half-space just outside the box, that moment might carry with it a 4.5% probability that a goal will be scored on the possession. He might then slip a ball between the fullback and the center back to an onrushing wide forward/winger type who is now free in on goal from a wide angle and upon receiving the ball now has a 15% chance of scoring on the possession (the pass/receipt collectively is worth 10.5%). The player, who we may as well call Thierry Henry at this point curls the ball inside the far post with his right foot, thus changing the probability of scoring from 15% to 100%. Technically this shot changed the goal scoring probability by 85% and could be valued as such. That same scenario where the player misses could be valued as a -15%, a complete possession loss in value.
But scoring this sequence by including the last change in probability from when the ball is struck to when it crosses the goal mouth is going to give us a bunch of accounting records for attacking players that we know are in medias res of regressing to the mean. If we take these accounting records and project forward the player’s contributions to future goal difference, unless he took 500 shots in the season, we are absolutely at the mercy of his short-term noisy finishing. The player who is currently running hot will be projected forward to run hot and contribute excessive goal difference, and the player running cold will project forward as actively destroying his team’s goal scoring probabilities (turning a bunch of 10% chances and 15% chances to zero. We know from basic analysis that neither of these trends will persist, so it’s best just to strip out the noise as a pro-forma adjustment by capping off the sequence the moment the shot is taken, effectively measuring a forward’s contribution based on his ability to increase his team’s chances of scoring by receiving the ball in the box and converting those receipts into shooting chances (for a striker, this ends up being similar to how traditional shot xG-based analysis would work).
ASA’s “Goals Added” model includes this pro-forma adjustment in it by design (opinions may differ as to whether it’s better this way, or whether the original accounting should be preserved, and then secondly adjusted). When a player takes a shot, g+ records the value of the action as follows: if the shot is off-target, it records the value of the shooting action as the difference between the shot-xG and the value of the possession before the shot. If the shot is on-target, it records that same value (shot xG minus pre-shot EPV) plus it adds to it an additional bonus (e.g. and additional 1% chance of scoring) representing the likelihood of the shot having been put on target being saved and the attacking team retaining possession and then scoring before turning it over. Skip this next sentence if you don’t care much about this stuff. Essentially, because the pre-shot possession value is “burdened” with the very small chance that a shot is taken, then saved, then collected as a rebound and then scored, if we cap our accounting such that the shooting player can only be rewarded the shot-xG and never attain this additional rebound value percentage, then on net shooting will load as a negative action, which it obviously is not.
The trouble with earning penalties
A clean EPV model might measure the value of drawing a foul in the box as the difference between the chance of scoring a penalty kick, typically 78%, and the value of the possession before the foul occurred in the box. If you take the above example, where Thierry Henry was slipped in 1v1 with the goalkeeper and had a 15% chance of scoring from a wide angle, if instead of shooting he was taken down via a slide tackle from behind, he would’ve earned a 78% penalty kick opportunity, and a true descriptive EPV model might assign him the +0.63 action of drawing a foul in the box (0.78 minus 0.15). And this would be an accurate description (i.e. accurate accounting) of the change in the team’s fortunes as a result of the foul being called. But if we’re going to take that accounting record, along with all of the player’s other records and try to project it into the future, that weighty +0.63 is going to overshadow so many actually predictive and recurring moments that a player like Thierry Henry generates to separate himself from other attackers (e.g. the receiving value he generates from tireless making runs in behind or the passing value he generates from seeing the field with a clear vision). Even if Henry repeatedly draws penalties well, there’s going to be some other player who’s going to get elbowed in the face at the corner of the box that will also earn a penalty, and he’ll look better than he really is in the data.
We’re better off nerfing this value a bit. And again here, this is something “Goals Added” does automatically. When a player is fouled in the box, g+ credits the player as if he had successfully dribbled by an opponent from that spot, a very valuable action in the penalty box, but not the overly punitive 0.6-0.7 type value-add event. We absolutely do want to project forward a player’s future contributions related to dribbling in the box, but this approach is less beholden to noise in the historical data.
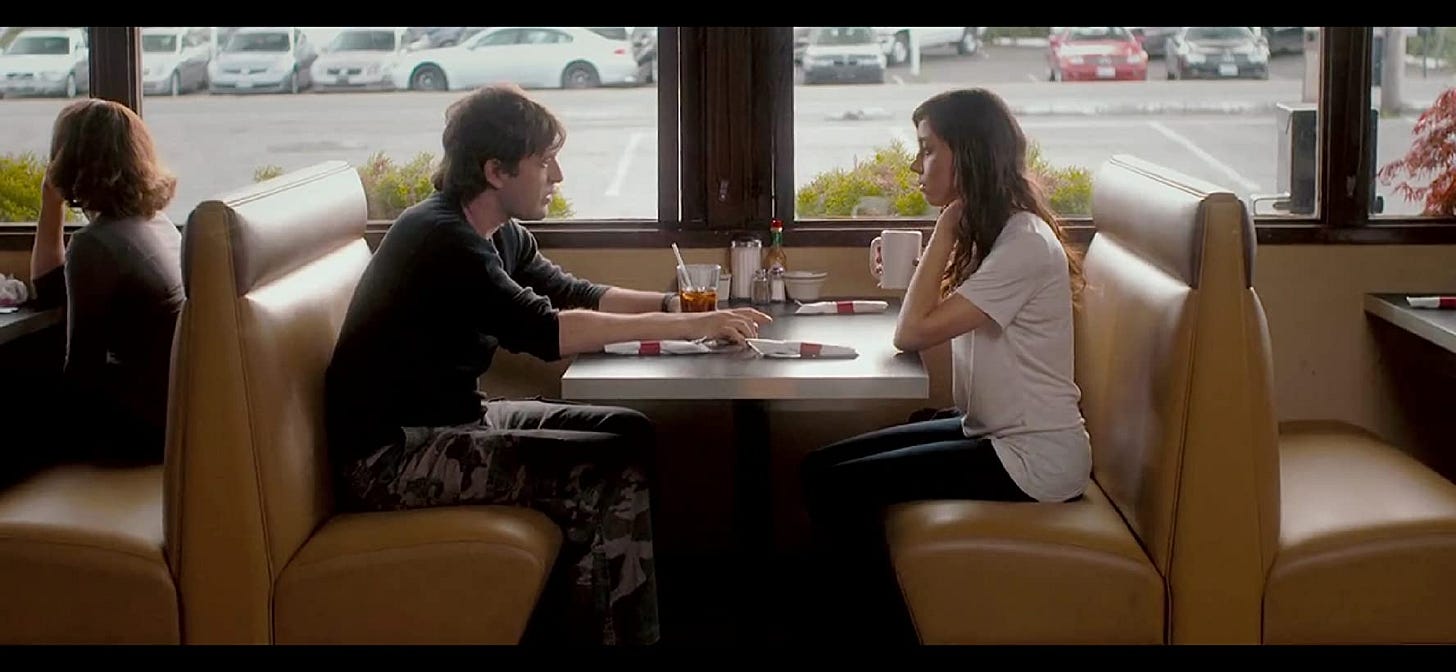
Up Next
I hope this post has been somewhat illustrative of some of the basic principles behind the difference between historical data and predictive metrics or even projected results. This list of examples is not all-inclusive, and I would encourage any player recruitment department to think holistically about its use of data and what makes for good projections and what is just noise. Any and all such adjustments are probably fair game as part of Step 2 before we move into what’s left here in the projection cycle (Steps 3-5) (and then move to benchmarking, and then onto the full strong-form functional system).
A note on where we go from here: taking historical data records and using them to predict the future is really hard. It’s helpful to take the step we took in this episode and adjust out noise, but after you do that, you’re still confronted with the most difficult of challenges that human beings face: uncertainty. Further complicating things is the fact that individual player contributions into a team’s output are not linear and they aren’t the only contributions. How best to theorize or design a model that articulates all of these interworking elements well is a really difficult question and a question that I cannot solve in this newsletter. It is an important question though, and one that any world class front office MUST attempt to solve, or else like what are you doing? For it to make any sense, a GM or sporting director has to hire to build a team of data analysts, performance analysts, scouts, coaches, consultants, economists(?) etc capable of generating the important insights needed to understand what drives competitive value at a football club, and then he must wisely fold them all together into a coherent model.
That is all to say, to borrow a poker expression, I do not have “the nuts” here. I have not “solved soccer,” and if I did I wouldn’t be writing these- I’d probably be placing big bets somewhere. But, I think the coming posts will be important in helping you all —you who are smarter than me, who have more experience than me, and who have better connections, who have a keener insight for the game, and who have the potential to build something really great — to get closer to doing so. If the posts to date (setting the scene by analogy and walking through the merits of event data, and bringing the financial valuation process to life a bit) were in my wheel house, and low-hanging fruit, then what’s to come is decidedly not that, but almost certainly more exciting. Stay tuned. It’s possible that for one more post, we’ll need to stop at the gas station and fill up again, tie off some loose ends before venturing out over the horizon.