Pit-stop: Odds & Ends
A glance at the road map and some assorted "Absolute Unit" relevant thoughts on EPVs
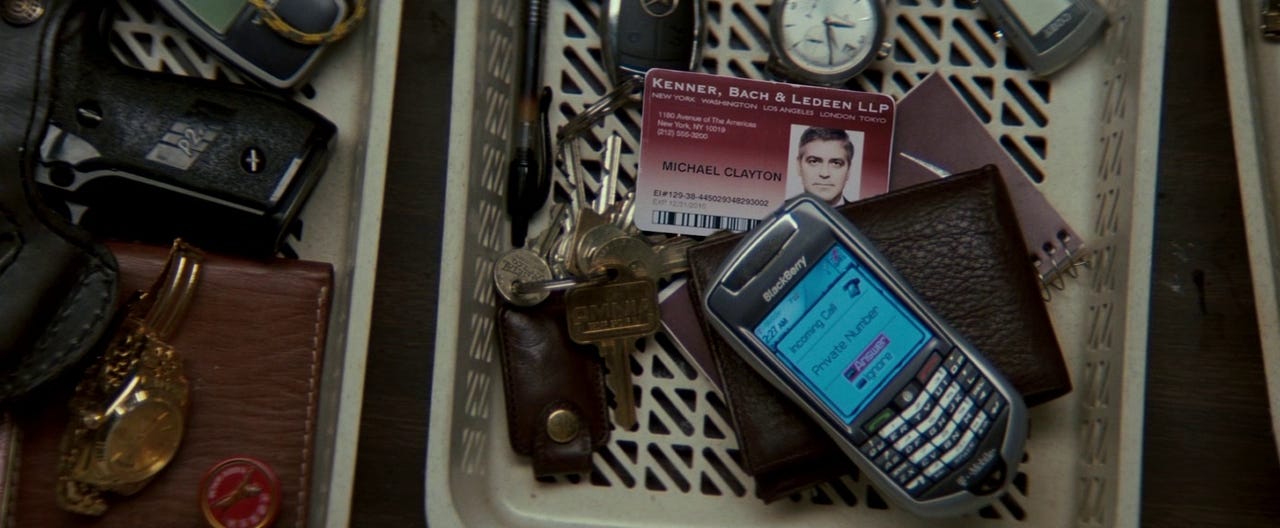
I mentioned at the tail end of last week’s post, as we march forward through the standing up of a soccer player evaluation process that mirrors a finance process, we are at a moment where we’ll need to jump from the more philosophical ponderings of the nature of soccer data as accounting records and toward the more difficult task of using all the available information and trying to predict future soccer stuff. Because again the idea (among other things) is to better structure the process by which the club puts together a squad of players with the intent of meeting future competitive operating goals, goals which we allege for several reasons should be denominated in a team “goal difference” target. We want to project a player’s future contribution toward that team target and then benchmark him against available options as a way to “value” a potential player contract first in terms of “marginal goal difference contribution,” and then after (many posts from now), we will need to translate things from the currency of goal difference to the currency of budget dollars since the operating constraint is budget dollars. I hashed together a quick snapshot of where we are below and where we’re going (though this is certainly not linear — I can’t tell you whether we’re halfway through, or more than halfway through, or less):
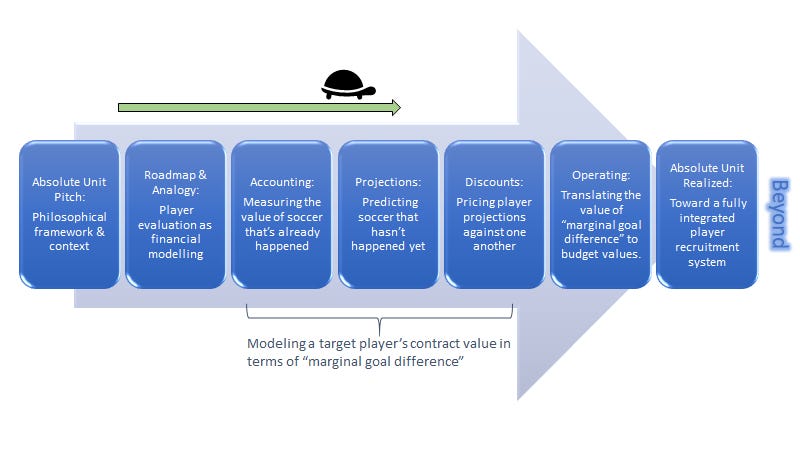
There’s plenty of stuff we should probably just check back to before we continue into the really hard stuff of "predicting the future” which in soccer involves trying to create a model of what drives “goal difference” value for a club - players, coaches, various activities, etc. This post doesn’t jump into that stuff. Just some odds and ends really.
On the “expected marginal goal difference” unit as more than a recruitment tool
The focus of this newsletter to date has been (and will continue to be for some time) on player recruitment, but the beauty of setting a measurable objective for the organization and then attaching to it the “universal units” used in key decision making is that the universality of the thing extends (duh) beyond player recruitment. I recently stumbled upon this quote from April of this year from Devin Pleuler, Director of Analytics at Toronto FC who was talking to Tom Bogert at MLS (emphasis added):
“I’m not actually a huge fan of expected goals as a standalone metric, but a lot of our metrics use expected goals as a unit or a currency… So, instead of looking at a game for this team had this many expected goals, we look at things on a more granular basis. We say, this pass increased our chances of scoring on this possession by this amount. That amount is measured in expected goals. But we don’t look at expected goals by itself.”
Sound familiar? It appears that we can add at least one other club to the list next to Liverpool and Barcelona who are clearly using an “EPV” or “non-shot xG” like model to assign units of account or currencies to the events of soccer matches (and probably for some time now). In Devin’s case here it looks like he’s talking about game analysis: a match report, or perhaps you might extend this to opposition analysis. And judging from another of his quotes from the same article, you can expect that if Toronto is breathing xG units into the raw data as part of their match analyses, they’re probably also doing this to support their recruitment efforts:
For me, player recruitment and the optimization of it is actually the place where analytics can make the largest amount of difference… I’d say it’s where I can provide 80 percent of my value.... Having an efficient scouting structure that allows data to cover huge swaths of the world, and doing due diligence faster with confidence, you can become a lot more efficient in the transfer market which means millions of dollars in value gains.”
The “fully operational battle station” that Absolute Unit ultimately advocates for includes this sort of seamless integration across all important club-generated insights. If you’re an analyst, you might mine the last two seasons of your own club’s data to identify the most frequent and most powerful g+ passes they generate. You might ask “does the coaching staff’s game model create certain types of high value sequences more than others (a question of play style perhaps)?” You would need these sequences in the data to be attached to units of account in order to sort/filter the match data this way. You might communicate to the coaching staff the sorts of sequences that are generating the highest values.
Then, when you go to target a new signing, you might be focused then not only on his total past contribution to his team’s expected goal difference based on all the actions he was involved in, but you might also filter to find a player with not only a suitable total, but who also plays plenty of the exact type of high value g+ passes that you identified in your team analysis as one of the primary attacking moves core to your team’s play style or identity, and then load up the film of his touches.
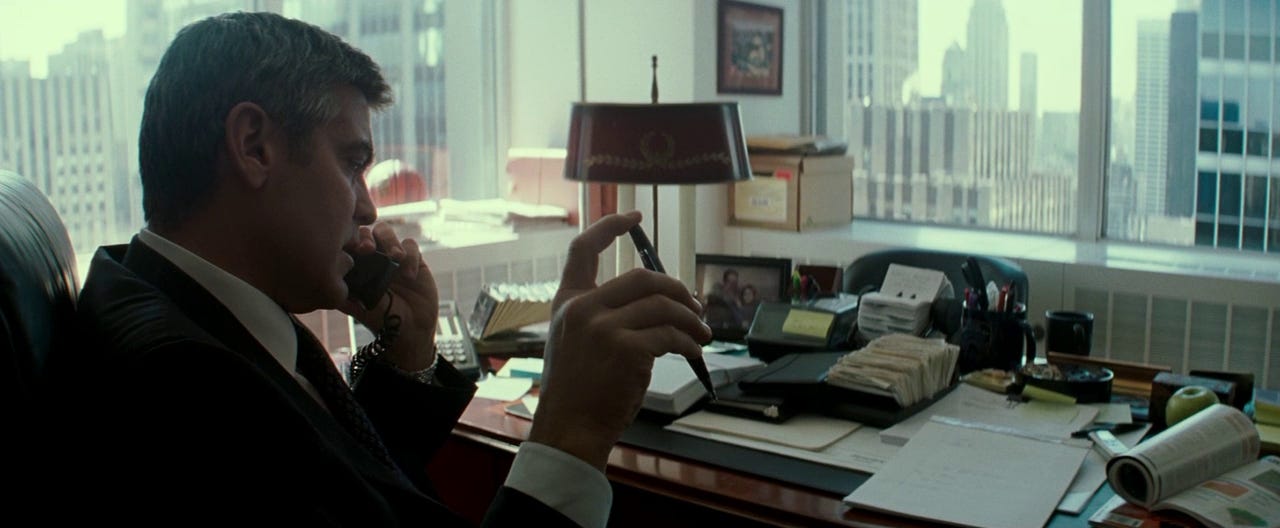
On qualitative data
In the last couple of chapters, when we’ve discussed the concept of “obtaining accounting records,” we have relied heavily on the actual data-side of things: the soccer event data (and an acknowledgment that tracking data will bring even more into this). The story has been that we have these nice, rich raw accounting records and they just need something like an EPV model to breathe a unit of value into them. But not all clubs have the same confidence in data and data analytics, and I made the case early that whether a club uses quantitative data or not, they should still read this site. They should still set an overall objective denominated in “goal difference” and then necessarily in order to organize their efforts towards that objective should project the contribution toward that target goal difference of various personnel decisions.
So this is partly just a reminder. While there’s been a ton of data analytics talk in the recent posts, theoretically you could be doing a similar exercise with a robust structure around your recruitment process where let’s say the scouting reports themselves (not the sea of event data) serve as “the accounting records.” You would still want to build up some sort of rigorous, repeatable process in order to infuse those accounting records with the unit of account of goal difference that your organization’s goals are set in (i.e. you would want to require a bottom line of each scouting report to be some sort of “+0.2 goal difference per 90” type score, even if there was no data-modeling behind it). This would be difficult, but perhaps a start would be training the scouts to grade more systematically, to avoid extremes, and to guard against biases (I’m sure much of this is already standard operating procedure). And the same “normalization adjustments” we walked through in the last post would still need to be applied consistently, whether that’s training your scouts to be mindful of these non-recurring events (e.g. penalties and set pieces), or having them separately score the target player in these different phases of the game, so you could quarantine the effects when you go to project forward from the scouting reports.
Even still, I would advocate for a club to “run the EPV on the event data” automatically regardless of whether they use this information to build up the player evaluation, and then just include a comparison of the two scores (the data and the scouting reports), at the step 1 accounting stage, and also at the end when the projections are completed (for the automated portions of the projection process - more to come). If you don’t want to rely on the data, that’s fine. But documenting the qualitative and the quantitative side by side might help when you go back and do retrospective reviews of your recruitment efforts. If the data is tracking closely with the scouting, that’s great, and possibly an opportunity to use the data to cover more ground and replicate your scouting efforts world wide. If not, there’s an opportunity to ask “why” and potentially improve the scouting, the data, or both.
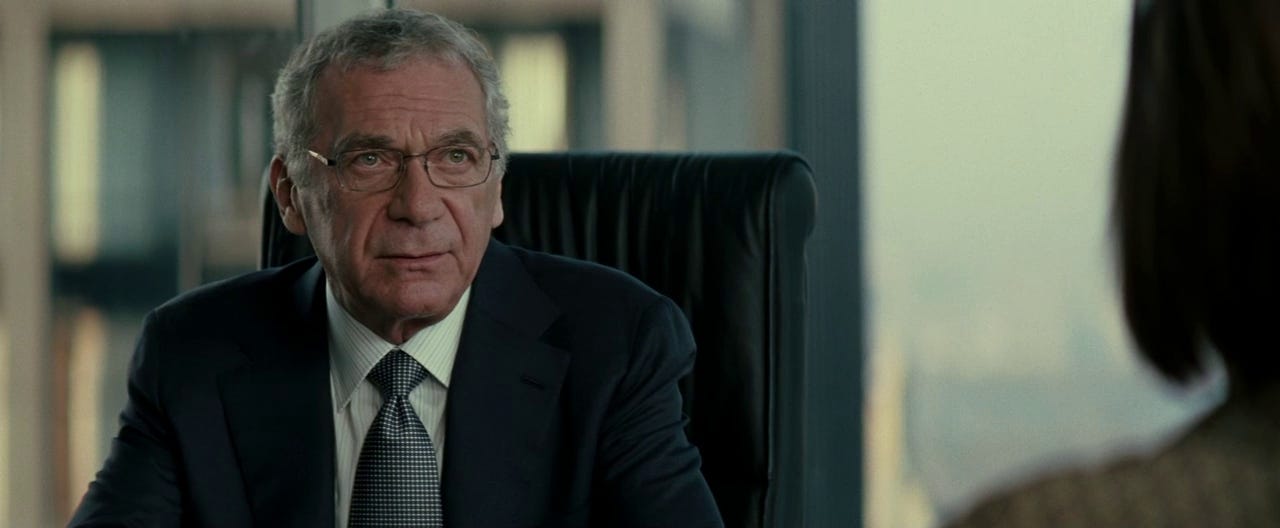
Expected Possession Value isn’t perfect
Before we move onto projections, I want to clarify something about “Step 1” and the accounting records. I made what I think is a central argument to the A-Unit premise that “Sure, soccer data is imperfect but so is financial accounting data, and the financial world doesn’t just throw its hands up and say ‘O well, data doesn’t really apply to financial modeling!’ so similarly, we shouldn’t let the imperfections of soccer data prevent the use of analytics in the sport.” In addition to the imperfections that exist in both sets of accounting records, I then acknowledged that soccer data has an additional issue that required remedying, which was the lack of a unit of value attached to each record the way financial accounting has this, and next we largely “solved” this by infusing the EPV model outputs into the records. I just want to clarify that this step of adding “value information” via EPV does not fix the natural imperfections that exist in soccer data (and in financial accounting), but rather it is a solution for the unit of account problem. So what’s left is still an imperfect accounting record, and that’s fine. I don’t think we should throw it out. I just want to emphasize that EPV isn’t some miracle cure to the imperfections or limitations in data.
Further, EPV models in the public sphere are very much still in progress and being improved. Recommending viewing on this is Thom Lawrence’s Statsbomb conference talk: “Some Things Aren’t Shots” where he talks through some of the interesting dilemmas.
So there’s that. I still think it’s an elegant solution for our “soccer event data as accounting records” problem statement, and it makes sense to me to move forward towards projections with this concept in place. Each club will need to assess whether to build this logic in house or contract for whichever available model they feel is most intuitive/strongest for their purposes. Further, each club’s mileage may vary with regards to supplementing this quantitative data (warts and all) with judgmental qualitative factors from scouts.
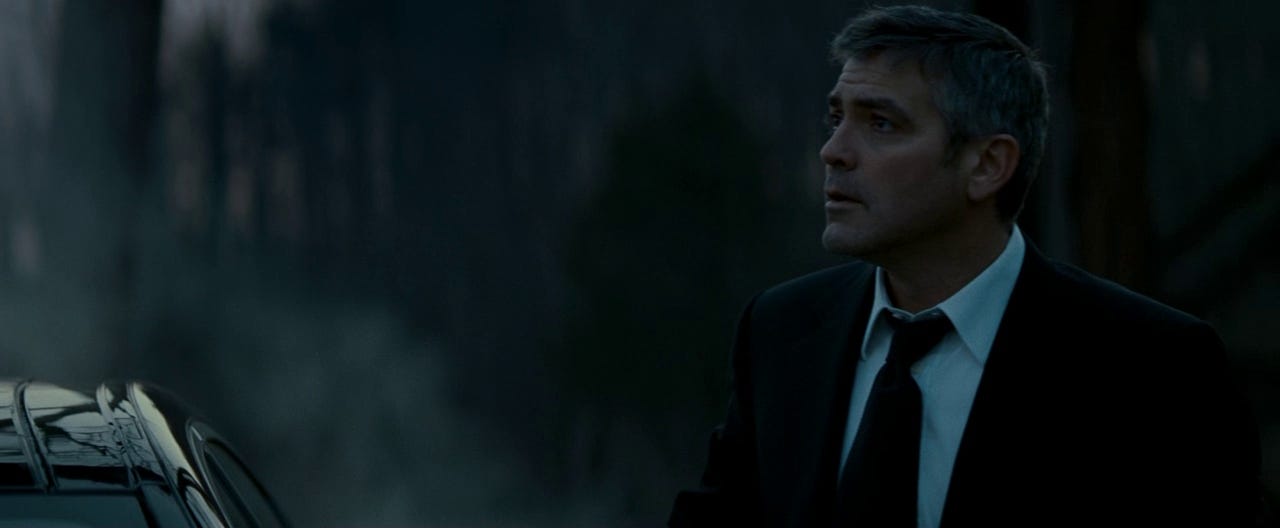
On ‘Wins above Replacement”
John Muller brought to my attention an article published last week by Ben Lindbergh at The Ringer summarizing a brief history of the development of the “Wins Above Replacement” (WAR) stat in baseball and how clubs thought about value in a time before that metric measured and articulated it so well. There are some good “absolute-unit-relevant” quotes in here, chief among them:
Before those stats existed, sabermetric godfather Bill James says, “We just really did not know, you know? Until we worked things out, we just had no concept of the scale of elements of the game.”
The scale of elements of the game. I am only vaguely familiar with Bill James writings and more familiar with his status as godfather of sports analytics, so it occurs to me there’s probably a lot about “WAR” that I need to catch upon, lest I accidentally stumble into pitfalls already navigated by James and other luminaries who sought to put an absolute unit on baseball. I’ll try to do some catchup homework there soon. But I love that quote because it’s a much clear-eyed articulation of what I was getting at before in an earlier post:
Clubs with good scouts and good analysts do generate good insights. To the extent the decision makers really internalize those insights and use them to sign players, that’s great and directionally, it’s a win. It’s likely sustainable. But even in an environment like that, I would allege there’s many a slip 'twixt the cup and the lip. The value of any given decision is not binary, in the sense that not all good decisions are equally good, nor are bad ones equally bad. This spectrum of values feels like it’s the first bit of information to be lost in the transfer from insight to decision if the correct question is not posed to begin with. The purpose of “Absolute Unit” then is to deploy a framework or interface such that the great insights that scouts, analysts, coaches, players etc generate are properly valued and connected to important decision making.
Anyhow, yea the “scale of the elements of the game.” Speaking of WAR, something we’ll touch on going forward is that as far as I know, squad construction in baseball based mostly on WAR or projected WAR “works” well because baseball is a series of individual duels/challenges where a player’s “execution” is largely independent of his teammates. While his “contribution” to team output might be a function of his own execution and also the execution of teammates near to him in the batting order that need to be on base for him to drive them in, and the execution of his other teammates further away in the batting order, who need to get hits to marginally increase the number of at-bats the player experiences, on the whole the modelling of converting projected “WAR” into team wins seems pretty clean.
The nature of soccer is obviously different. I do see talk in some corners of the soccer analytics community of seeing the game as a series of individual “duels,” but I really don’t see it this way as a general observation. And so to crack the “projection” problem, we’ll have to do some analysis of what creates success for a team, and make some assumptions about the relative contribution of different factors and activities. There are much more qualified people to generate these estimates and/or support the relevant assumptions, but we can at the very least frame them up, and take a shot as an illustration. So that’s coming soon.